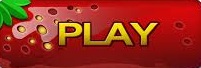
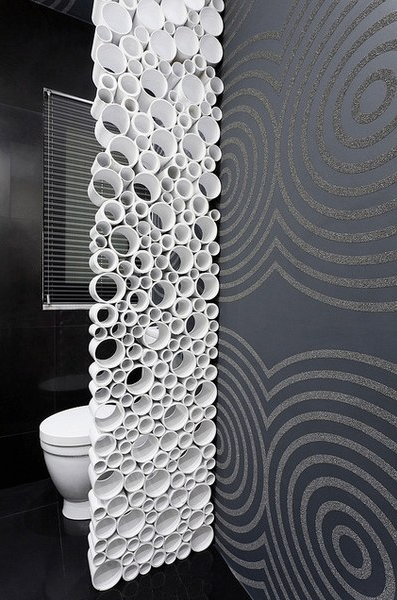
Some variables (eg., precipitation) may have little or no spatial correlation at high frequency sampling (eg., 3-hourly) but on monthly, seasonal or annual mean time scales may be 'reasonably' smooth is space. Most geophysical variables ( eg., temperature) are highly spatially correlated at all time scales. The choice of which method to use should be guided by a combination of the intended use of the interpolated data and the structure of the original variable. Which Spatial Interpolation Method Should be used? This is a very fast operation and is ideally suited to processing a large number of grids. Finally, the user can read the generated weights and perform a 'sparse matrix multiply' to effect the regridding. Depending upon the grid structures and the sizes, this may take a significant amount of time due to searching requirements.
#GRIDDED MATTING SOFTWARE#
The software then generates a netCDF file which contains an array of weights. Initially, the user specifies the type of interpolation to be performed ( eg, bilinear, conservative or patch) and the source and destination grid spatial coordinates. Some interpolation schemes use a multi-step approach. However, curvilinear and unstructured grids may involve sophisticated searching algorithms to determine the points surrounding the location to be interpolated.
.tif.jpg)
Virtually all data processing tools provide a function to implement some form of bilinear interpolation for rectilinear grids. The bilinear method is easy to program and apply when the source and destination grids are rectilinear. The most commonly used methods of climate grid interpolation are bilinear, conservative and patch. Certain applications of regridded data may necessitate two specialized interpolation methods to achieve required physical ( eg, flux conservation) or mathematical ( eg, higher order derivatives) requirements: conservative and patch. Classic interpolation methods include: bilinear, nearest neighbor, inverse distance, spline, binning, spectral and triangulation. Quantitative evaluation of data contained on different grids requires regridding to a common grid. Why the variety of grids? Reasons include advances in computer capabilities, computational efficiency, addressing pole singularities and physical constraints. For our purposes, grids described by one-dimensional latitude and longitude coordinates () will be called rectilinear grids where the coordinates are two-dimensional () will be called curvilinear and, grids in which the grid coordinates require a list of nodes ( connectivity information) will be called unstructured.
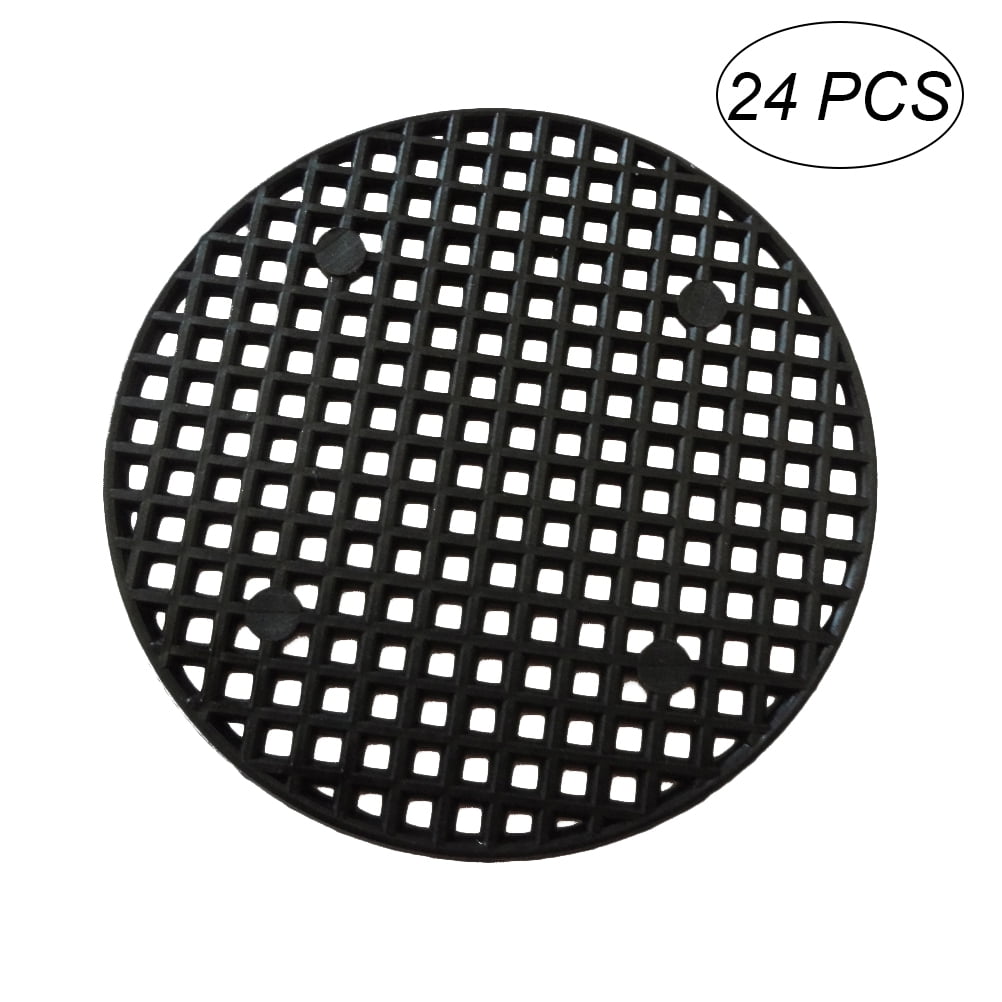
Grids used in climate research fall into several categories: regular, rectilinear, curvilinear and unstructured. Further, most climate grids are georeferenced on a sphere where pole singularities and the convergence of the longitude meridions can be issues that need to be addressed. Using an inappropriate interpolation scheme may lead to misleading results. There are numerous grid interpolation methods and users should choose the method appropriate for the intended task. However, most commonly, regridding refers to spatial interpolation. This could involve temporal, vertical or spatial ('horizontal') interpolations. Regridding is the process of interpolating from one grid resolution to a different grid resolution.
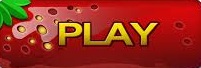